Using machine learning to design better catalysts
by Nicola Nosengo, EPFL, NCCR MARVEL
A project by the COSMO Lab at EPFL in collaboration with the chemical company BASF, on a computational study of high-entropy alloys (HEAs) was one of the highlights presented during the company’s traditional end-of-the-year press conference.
The project focusses on exploring the potential of HEAs to reduce the quantity of precious metals used in catalysts. Specifically, the EPFL group used machine-learning to accelerate the search for candidate HLAs for catalysis applications, training a model that can describe up to 25 transition metals to study the tendency of different elements to segregate at the surface of a HEA. Published as a preprint in October, the study builds on a 2018 publication by the same group – led by Michele Ceriotti and part of NCCR MARVEL - that introduced a a general-purpose ML model for the study of HEAs and applied it to crystalline bulk phases.
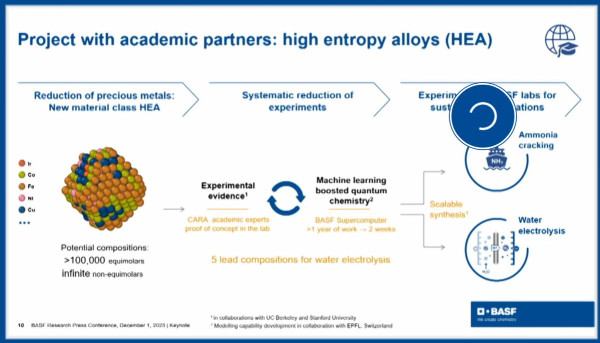
Low-volume newsletters, targeted to the scientific and industrial communities.
Subscribe to our newsletter