Ksenia Briling
Interview by Carey Sargent, EPFL, NCCR MARVEL, February 2023
Why are you a scientist? / Why did you choose a scientific career?
I think I’ve wanted to be a scientist since I was 15 or 16. Before that I wanted to be a civil engineer like my parents, and then for some time I wanted to be a writer, but I don’t write very well! First I liked the natural sciences more than other sciences, then I started participating in chemistry olympiads – haven’t gotten to the International Chemistry Olympiad though – and it kind of defined my path into the department of chemistry at MSU, which is mainly focused on fundamental research. And then, after a few years of doing it, I couldn’t imagine my life doing something else.
What motivates you?
First of all, I enjoy it. It is interesting. Then, I like to work on developing tools or methods that could be eventually used by other researchers. It hasn’t happened yet, but one of my goals it to make something useful for others.
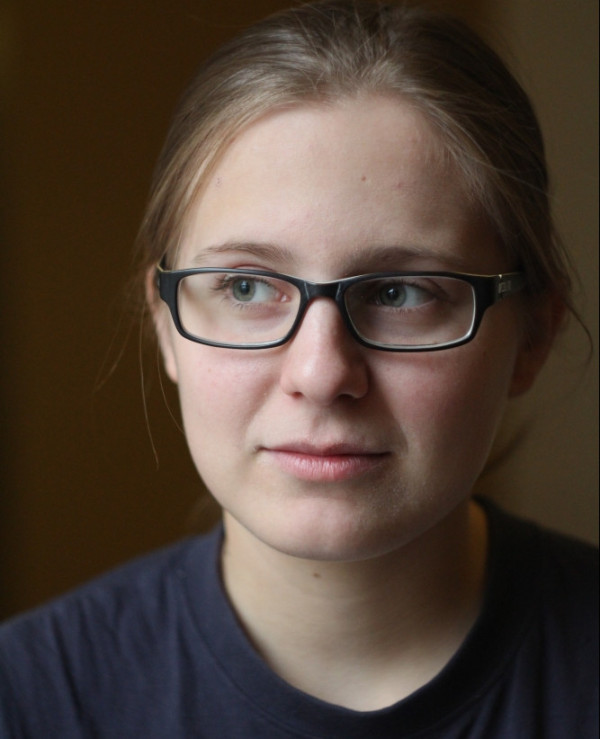
What’s the aim of your research in general?
We are working on merging quantum chemistry and machine learning. I am working on creating new molecular representations for use in machine learning. Quantum chemists usually work with 3D structures of molecules. To feed a structure into a statistical model, you need to encode it into a vector and this is not a trivial task. Most representations use only the geometrical structure and then the model ignores everything else about the molecule, such as charge or spin. We, but not only us, are working on having some information about the distribution of electrons in the molecule also encoded in the representation. This improves the quality of learning of the different properties.
Do you think women face particular challenges in science?
I’d say that women face particular challenges, but it’s not specific to science. It’s good that now we have more role models, like female PIs, that can show little girls that there is a path in science for us. But this won’t help if at some point you’re forced to choose between your job and your family. And I think it can’t be solved when family works like it works now, when the whole society works like it works now.
Which papers are you most proud of?
One is an old paper with my master supervisor on initial guesses for quantum chemistry (https://link.springer.com/article/10.1007/s00214-019-2521-3). It’s short, but I like what we did and it could be useful.
Another one is Impact of quantum-chemical metrics on the machine learning prediction of electron density, where we take a model that our lab had developed in cooperation with COSMO and improved it by finding a way to include more physics into the machine learning.
Another one’s SPA HM: the spectrum of approximated Hamiltonian matrices representations, where we contributed to the field of molecular representations. We took a very simple quantum chemical property from a very simple model, and it worked as a pretty good descriptor for machine learning, which we didn’t even expect.