D&D1 - Organic Crystals
Group Leaders
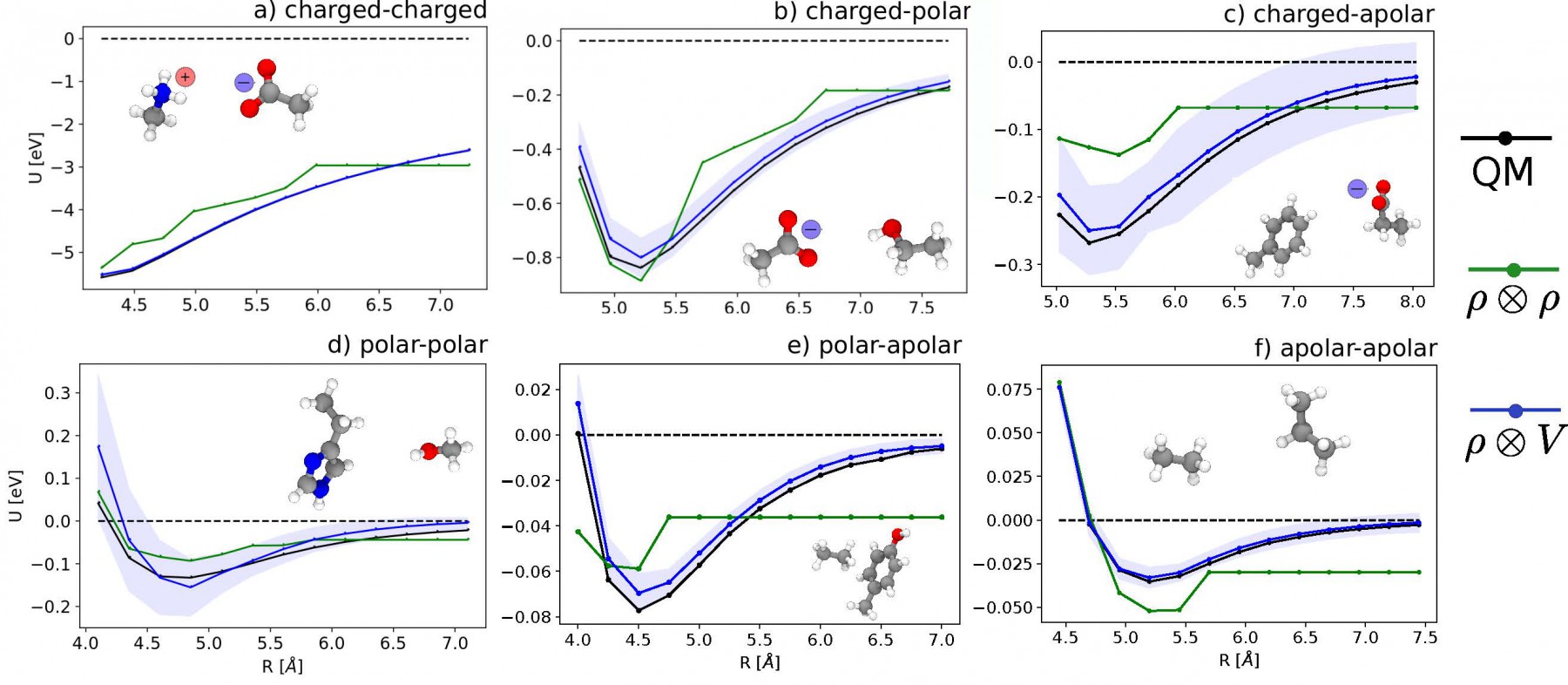
Median-error binding curves for six different classes of intermolecular interactions. (Black lines) quantum-mechanical calculations. (Green lines) predictions of a conventional ML model, that relies entirely on short-range interactions. (Blue lines) multi-scale LODE model, that combine short-range information with an asymptotic term mimicking multipole electrostatics [1].
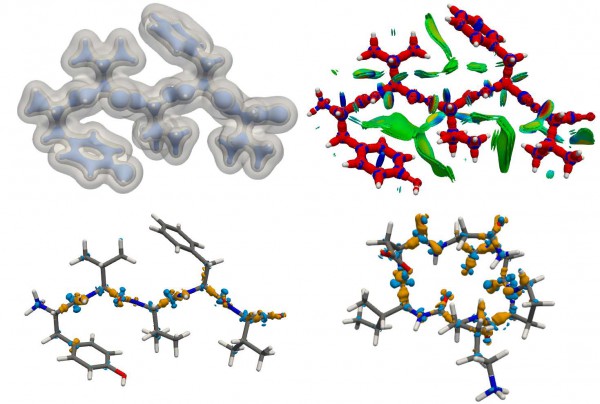
Machine-learned prediction of the charge density of the peptide encephalin [2]. The charge is sufficiently accurate to capture the fine details of the charge density that are associated with covalent and non-covalent interactions, as identified by the DORI fingerprint. The error (shown in the bottom panels for enkephalin and a cyclopeptide) concentrates in the region of the peptide bond, as the training set contains no examples of such chemical feature.
[1] Grisafi-arxiv-2020 A. Grisafi, J. Nigam, and M. Ceriotti, Multi-scale approach for the prediction of atomic scale properties, arXiv:2008.12122 (2020). [Open Access URL]
[2] A. Fabrizio, A. Grisafi, B. Meyer, M. Ceriotti, C. Corminboeuf, Electron density learning of non-covalent systems, Chemical Science 10, 9424 (2019). [Open Access URL]
Related publications (until January 2023)
- M. Balodis, M. Cordova, A. Hofstetter, G. M. Day, L. Emsley, De Novo Crystal Structure Determination from Machine Learned Chemical Shifts, Journal of the American Chemical Society 144, 7215 (2022). [Open Access URL]
Group(s): Emsley / Project(s): DD1 - M. Cordova, P. Moutzouri, B. Simões de Almeida, D. Torodii, L. Emsley, Pure Isotropic Proton NMR Spectra in Solids using Deep Learning, Angewandte Chemie International Edition 2023, e202216607 (2023). [Open Access URL]
Dataset on Materials Cloud.
Group(s): Emsley / Project(s): DD1 - M. Cordova, E. A. Engel, A. Stefaniuk, F. Paruzzo, A. Hofstetter, M. Ceriotti, L. Emsley, A Machine Learning Model of Chemical Shifts for Chemically and Structurally Diverse Molecular Solids, The Journal of Physical Chemistry C 126, 16710–16720 (2022). [Open Access URL]
Dataset on Materials Cloud.
Group(s): Ceriotti, Emsley / Project(s): DD1 - V. Kapil, E. A. Engel, A complete description of thermodynamic stabilities of molecular crystals, Proceedings of the National Academy of Science of the USA 119, e2111769119 (2022). [Open Access URL]
Dataset on Materials Cloud.
Group(s): Ceriotti / Project(s): DD1 - C. W. Myung, B. Hirshberg, M. Parrinello, Prediction of a Supersolid Phase in High-Pressure Deuterium, Physical Review Letters 128, 045301 (2022). [Open Access URL]
Dataset on GitHub.
Group(s): Parrinello / Project(s): DD1 - B. Parsaeifard, S. Goedecker, Manifolds of quasi-constant SOAP and ACSF fingerprints and the resulting failure to machine learn four-body interactions, The Journal of Chemical Physics 156, 034302 (2022). [Open Access URL]
Group(s): Goedecker / Project(s): DD1 - M. Thürlemann, L. Böselt, S. Riniker, Regularized by Physics: Graph Neural Network Parametrized Potentials for the Description of Intermolecular Interactions, arXiv:2206.00355 (2022). [Open Access URL]
Dataset on ETHZ Research Collection.
Group(s): Riniker / Project(s): DD1 - M. Thürlemann, L. Böselt, S. Riniker, Learning Atomic Multipoles: Prediction of the Electrostatic Potential with Equivariant Graph Neural Networks, Journal of Chemical Theory and Computation 18, 1701 (2022). [Open Access URL]
Dataset on ETHZ Research Collection.
Group(s): Riniker / Project(s): DD1 - J. Nigam, M. J. Willatt, M. Ceriotti, Equivariant representations for molecular Hamiltonians and N-center atomic-scale properties, The Journal of Chemical Physics 156, 014115 (2022).
Dataset on Materials Cloud.
Group(s): Ceriotti / Project(s): DD1
- T. Karmakar, M. Invernizzi, V. Rizzi, M. Parrinello, Collective variables for the study of crystallisation, Molecular Physics 119, e1893848 (2021). [Open Access URL]
Dataset on PLUMED-NEST.
Group(s): Parrinello / Project(s): DD1 - S. N. Pozdnyakov, L. Zhang, C. Ortner, G. Csányi, M. Ceriotti, Local invertibility and sensitivity of atomic structure-feature mappings, Open Research Europe 1, 126 (2021).
Dataset on Materials Cloud.
Group(s): Ceriotti / Project(s): DD1 - A. Goscinski, F. Musil, S. Pozdnyakov, J. Nigam, M. Ceriotti, Optimal radial basis for density-based atomic representations, The Journal of Chemical Physics 155, 104106 (2021).
Group(s): Ceriotti / Project(s): DD1 - E. A. Engel, V. Kapil, M. Ceriotti, Importance of Nuclear Quantum Effects for NMR Crystallography, The Journal of Physical Chemistry Letters 12, 7701–7707 (2021).
Dataset on Materials Cloud.
Group(s): Ceriotti / Project(s): DD1 - D. Mandelli, M. Parrinello, A modified nudged elastic band algorithm with adaptive spring lengths, The Journal of Chemical Physics 155, 074103 (2021). [Open Access URL]
Group(s): Parrinello / Project(s): DD1 - M. Yang, T. Karmakar, M. Parrinello, Liquid-Liquid Critical Point in Phosphorus, Physical Review Letters 127, 080603 (2021). [Open Access URL]
Group(s): Parrinello / Project(s): DD1 - L. Bonati, Training collective variables for enhanced sampling via neural networks based discriminant analysis, Nuovo Cimento C 44, 125 (2021). [Open Access URL]
Dataset on PLUMED-NEST.
Group(s): Parrinello / Project(s): DD1 - K. R. Briling, A. Fabrizio, C. Corminboeuf, Impact of quantum-chemical metrics on the machine learning prediction of electron density, The Journal of Chemical Physics 155, 024107 (2021). [Open Access URL]
Dataset on Materials Cloud.
Group(s): Corminboeuf / Project(s): DD1 - M. Invernizzi, OPES: On-the-fly Probability Enhanced Sampling method, Nuovo Cimento C 44, 112 (2021). [Open Access URL]
Dataset on PLUMED-NEST.
Group(s): Parrinello / Project(s): DD1 - F. Musil, A. Grisafi, A. P. Bartók, C. Ortner, G. Csányi, M. Ceriotti, Physics-Inspired Structural Representations for Molecules and Materials, Chemical Reviews 121, 9759–9815 (2021).
Group(s): Ceriotti / Project(s): DD1 - S. Vela, A. Fabrizio, K. R. Briling, C. Corminboeuf, Learning the Exciton Properties of Azo-dyes, The Journal of Physical Chemistry Letters 12, 5957–5962 (2021). [Open Access URL]
Dataset on Materials Cloud.
Group(s): Corminboeuf / Project(s): DD1 - P. Ahlawat, A. Hinderhofer, E. A. Alharbi, H. Lu, A. Ummadisingu, H. Niu, M. Invernizzi, S. M. Zakeeruddin, M. I. Dar, F. Schreiber, A. Hagfeldt, M. Grätzel, U. Rothlisberger, M. Parrinello, A combined molecular dynamics and experimental study of two-step process enabling low-temperature formation of phase-pure alpha-FAPbI(3), Science Advances 7, eabe3326 (2021). [Open Access URL]
Group(s): Parrinello / Project(s): DD1 - A. Goscinski, G. Fraux, G. Imbalzano, M. Ceriotti, The role of feature space in atomistic learning, Machine Learning: Science and Technology 2, 025028 (2021).
Dataset on GitHub.
Group(s): Ceriotti / Project(s): DD1 - J. Lan, V. Kapil, P. Gasparotto, M. Ceriotti, M. Iannuzzi, V. V. Rybkin, Simulating the ghost: quantum dynamics of the solvated electron, Nature Communications 12, 766 (2021). [Open Access URL]
Dataset on Materials Cloud.
Group(s): Ceriotti, Hutter / Project(s): DD1, DD4 - L. Böselt, M. Thürlemann, S. Riniker, Machine Learning in QM/MM Molecular Dynamics Simulations of Condensed-Phase Systems, Journal of Chemical Theory and Computation 17, 2641 (2021). [Open Access URL]
Dataset ETHZ Research Collection.
Group(s): Riniker / Project(s): DD1 - M. Cordova, M. Balodis, B. Simões de Almeida, M. Ceriotti, L. Emsley, Bayesian probabilistic assignment of chemical shifts in organic solids, Science Advances 7, eabk2341 (2021). [Open Access URL]
Dataset on Materials Cloud.
Group(s): Ceriotti, Emsley / Project(s): DD1 - E. A. Engel, Identification of synthesisable crystalline phases of water - a prototype for the challenges of computational materials design, CrystEngComm 23, 252–263 (2021). [Open Access URL]
Group(s): Ceriotti / Project(s): DD1 - A. Fabrizio, K. R. Briling, C. Corminboeuf, SPAHM: the Spectrum of Approximated Hamiltonian Matrices representations, arXiv:2110.13037 (2021). [Open Access URL]
Dataset on Materials Cloud.
Group(s): Corminboeuf / Project(s): DD1 - A. Grisafi, J. Nigam, M. Ceriotti, Multi-scale approach for the prediction of atomic scale properties, Chemical Science 12, 2078–2090 (2021). [Open Access URL]
Dataset on Materials Cloud.
Group(s): Ceriotti / Project(s): DD1 - T. W. Ko, J. A. Finkler, S. Goedecker, J. Behler, A fourth-generation high-dimensional neural network potential with accurate electrostatics including non-local charge transfer, Nature Communications 12, 398 (2021). [Open Access URL]
Dataset on Materials Cloud.
Group(s): Goedecker / Project(s): DD1 - B. Parsaeifard, D. S. De, J. A. Finkler, S. Goedecker, Fingerprint-Based Detection of Non-Local Effects in the Electronic Structure of a Simple Single Component Covalent System, Condensed Matter 6, 9 (2021). [Open Access URL]
Dataset on Materials Cloud.
Group(s): Goedecker / Project(s): DD1 - B. Parsaeifard, D. S. De, A. S. Christensen, F. A. Faber, E. Kocer, S. De, J. Behler, O. A. von Lilienfeld, S. Goedecker, An assessment of the structural resolution of various fingerprints commonly used in machine learning, Machine Learning: Science and Technology 2, 015018 (2021). [Open Access URL]
Dataset on Materials Cloud.
Group(s): Goedecker, von Lilienfeld / Project(s): DD1, INC2 - V. Rizzi, L. Bonati, N. Ansari, M. Parrinello, The role of water in host-guest interaction, Nature Communications 12, 93 (2021). [Open Access URL]
Dataset on Materials Cloud.
Group(s): Parrinello / Project(s): DD1 - M. Yang, L. Bonati, D. Polino, M. Parrinello, Using metadynamics to build neural network potentials for reactive events: the case of urea decomposition in water, Catalysis Today (2021). [Open Access URL]
Dataset on Materials Cloud.
Group(s): Parrinello / Project(s): DD1 - B. Parsaeifard, D. Tomerini, D. S. De, S. Goedecker, Maximum volume simplex method for automatic selection and classification of atomic environments and environment descriptor compression, The Journal of Chemical Physics 153, 214104 (2020). [Open Access URL]
Dataset on Materials Cloud.
Group(s): Goedecker / Project(s): DD1 - G. Fraux, R. Cersonsky, M. Ceriotti, Chemiscope: interactive structure-property explorer for materials and molecules, Journal of Open Source Software 5, 2117 (2020). [Open Access URL]
Dataset on Zenodo.
Group(s): Ceriotti / Project(s): DD1 - D. Mandelli, B. Hirshberg, M. Parrinello, Metadynamics of Paths, Physical Review Letters 125, 026001 (2020). [Open Access URL]
Group(s): Parrinello / Project(s): DD1 - M. Veit, D. M. Wilkins, Y. Yang, R. A. DiStasio, M. Ceriotti, Predicting molecular dipole moments by combining atomic partial charges and atomic dipoles, The Journal of Chemical Physics 153, 024113 (2020). [Open Access URL]
Dataset on Materials Cloud.
Group(s): Ceriotti / Project(s): DD1 - N. Ansari, T. Karmakar, M. Parrinello, Molecular Mechanism of Gas Solubility in Liquid: Constant Chemical Potential Molecular Dynamics Simulations, Journal of Chemical Theory and Computation 16, 5279 (2020). [Open Access URL]
Dataset on Materials Cloud.
Group(s): Parrinello / Project(s): DD1 - D. S. De, B. Schaefer, B. von Issendorff, S. Goedecker, Nonexistence of the decahedral Si20H20 cage: Levinthal's paradox revisited, Physical Review B 101, 214303 (2020). [Open Access URL]
Group(s): Goedecker / Project(s): DD1 - K. Rossi, V. Jurásková, R. Wischert, L. Garel, C. Corminbœuf, M. Ceriotti, Simulating Solvation and Acidity in Complex Mixtures with First-Principles Accuracy: The Case of CH3SO3H and H2O2 in Phenol, Journal of Chemical Theory and Computation 16, 5139 (2020). [Open Access URL]
Dataset on Materials Cloud.
Group(s): Ceriotti, Corminboeuf / Project(s): DD1 - B. Hirshberg, M. Invernizzi, M. Parrinello, Path integral molecular dynamics for fermions: alleviating the sign problem with the Bogoliubov inequality, Chemical Physics 152, 171102 (2020). [Open Access URL]
Dataset on GitHub.
Group(s): Parrinello / Project(s): DD1 - L. Bonati, V. Rizzi, M. Parrinello, Data-Driven Collective Variables for Enhanced Sampling, The Journal of Physical Chemistry Letters 11, 2998 (2020). [Open Access URL]
Dataset on Materials Cloud.
Group(s): Parrinello / Project(s): DD1 - A. Fabrizio, K. R. Briling, A. Grisafi, C. Corminboeuf, Learning (from) the Electron Density: Transferability, Conformational and Chemical Diversity, CHIMIA 74, 232–236 (2020). [Open Access URL]
Dataset on Materials Cloud.
Group(s): Ceriotti, Corminboeuf / Project(s): DD1 - A. Fabrizio, B. Meyer, C. Corminboeuf, Machine learning models of the energy curvature vs particle number for optimal tuning of long-range corrected functionals, The Journal of Chemical Physics 152, 154103 (2020). [Open Access URL]
Dataset on Materials Cloud.
Group(s): Corminboeuf / Project(s): DD1 - A. Fabrizio, R. Petraglia, C. Corminboeuf, Balancing Density Functional Theory Interaction Energies in Charged Dimers Precursors to Organic Semiconductors, Journal of Chemical Theory and Computation 16, 3530–3542 (2020). [Open Access URL]
Dataset on Materials Cloud.
Group(s): Corminboeuf / Project(s): DD1 - J. A. Finkler, S. Goedecker, Funnel hopping Monte Carlo: An efficient method to overcome broken ergodicity, The Journal of Chemical Physics 152, 164106 (2020). [Open Access URL]
Group(s): Goedecker / Project(s): DD1 - D. Polino, E. Grifoni, R. Rousseau, M. Parrinello, How Collective Phenomena Impact CO2 Reactivity and Speciation in Different Media, The Journal of Physical Chemistry A 124, 3963–3975 (2020). [Open Access URL]
Group(s): Parrinello / Project(s): DD1 - R. Fabregat, A. Fabrizio, B. Meyer, D. Hollas, C. Corminboeuf, Hamiltonian-Reservoir Replica Exchange and Machine Learning Potentials for Computational Organic Chemistry, Journal of Chemical Theory and Computation 16, 3084–3094 (2020). [Open Access URL]
Dataset on Materials Cloud.
Group(s): Corminboeuf / Project(s): DD1 - M. Invernizzi, M. Parrinello, Rethinking Metadynamics: from Bias Potentials to Probability Distributions, The Journal of Physical Chemistry Letters 11, 2731 (2020). [Open Access URL]
Dataset on Materials Cloud.
Group(s): Parrinello / Project(s): DD1 - V. Kapil, D. M. Wilkins, J. Lan, M. Ceriotti, Inexpensive modeling of quantum dynamics using path integral generalized Langevin equation thermostats, The Journal of Chemical Physics 152, 124104 (2020). [Open Access URL]
Dataset on Materials Cloud.
Group(s): Ceriotti / Project(s): DD1 - M. Asgari, R. Semino, P. A. Schouwink, I. Kochetygov, J. Tarver, O. Trukhina, R. Krishna, C. M. Brown, M. Ceriotti, W. L. Queen, Understanding How Ligand Functionalization Influences CO2 and N2 Adsorption in a Sodalite Metal-Organic Framework, Chemistry of Materials 32, 1526 (2020).
1st dataset on CSD / 2nd dataset on CSD / 3rd dataset on CSD / 4th dataset on CSD / 5th dataset on CSD / 6th dataset on CSD / 7th dataset on CSD.
Group(s): Ceriotti, Queen / Project(s): DD1, PP7 - G. Csányi, M. J. Willatt, M. Ceriotti, Machine-Learning of Atomic-Scale Properties Based on Physical Principles, in Machine Learning Meets Quantum Physics, K. T. Schütt, S. Chmiela, O. A. von Lilienfeld, A. Tkatchenko, K. Tsuda, and K.-R. Müller, eds. (Springer International Publishing, Cham, 2020), vol. 968 of Lecture Notes in Physics, pp. 99–127.
Group(s): Ceriotti / Project(s): DD1 - T. Dornheim, M. Invernizzi, J. Vorberger, B. Hirshberg, Attenuating the fermion sign problem in path integral Monte Carlo simulations using the Bogoliubov inequality and thermodynamic integration, The Journal of Chemical Physics 153, 234104 (2020). [Open Access URL]
Group(s): Parrinello / Project(s): DD1 - I. Errea, F. Belli, L. Monacelli, A. Sanna, T. Koretsune, T. Tadano, R. Bianco, M. Calandra, R. Arita, F. Mauri, J. Flores-Livas, Quantum crystal structure in the 250-kelvin superconducting lanthanum hydride, Nature 578, 66 (2020). [Open Access URL]
Group(s): Goedecker / Project(s): DD1 - A. Fabrizio, K. R. Briling, D. D. Girardier, C. Corminboeuf, Learning on-top: regressing the on-top pair density for real-space visualization of electron correlation, The Journal of Chemical Physics 153, 204111 (2020). [Open Access URL]
Dataset on Materials Cloud.
Group(s): Corminboeuf / Project(s): DD1 - G. Fisicaro, S. Filice, S. Scalese, G. Compagnini, R. Reitano, L. Genovese, S. Goedecker, I. Deretzis, A. La Magna, Wet Environment Effects for Ethanol and Water Adsorption on Anatase TiO2 (101) Surfaces, The Journal of Physical Chemistry C 124, 2406 (2020). [Open Access URL]
Group(s): Goedecker / Project(s): DD1 - J. A. Flores-Livas, Crystal structure prediction of magnetic materials, Journal of Physics: Condensed Matter 32, 294002 (2020).
Group(s): Goedecker / Project(s): DD1 - J. A. Flores-Livas, L. Boeri, A. Sanna, G. Profeta, R. Arita, M. Eremets, A perspective on conventional high-temperature superconductors at high pressure: Methods and materials, Physics Reports 856, 1 (2020). [Open Access URL]
Group(s): Goedecker / Project(s): DD1 - M. Invernizzi, P. M. Piaggi, M. Parrinello, A Unified Approach to Enhanced Sampling, Physical Review X 10, 041034 (2020). [Open Access URL]
Dataset on Materials Cloud.
Group(s): Parrinello / Project(s): DD1 - H. Niu, L. Bonati, P. M. Piaggi, M. Parrinello, Ab initio phase diagram and nucleation of gallium, Nature Communications 11, 2654 (2020). [Open Access URL]
Dataset on Materials Cloud.
Group(s): Parrinello / Project(s): DD1 - M. D. Wodrich, A. Fabrizio, B. Meyer, C. Corminboeuf, Data-powered augmented volcano plots for homogeneous catalysis, Chemical Science 16, 12070–12080 (2020). [Open Access URL]
Dataset on Materials Cloud.
Group(s): Corminboeuf / Project(s): DD1 - A. Fabrizio, B. Meyer, R. Fabregat, C. Corminboeuf, Quantum Chemistry Meets Machine Learning, CHIMIA 73, 983 (2019).
Group(s): Corminboeuf / Project(s): DD1 - F. Musil, M. Ceriotti, Machine Learning at the Atomic Scale, CHIMIA 73, 972 (2019).
Group(s): Ceriotti / Project(s): DD1 - D. S. De, M. Krummenacher, B. Schaefer, S. Goedecker, Finding Reaction Pathways with Optimal Atomic Index Mappings, Physical Review Letters 123, 206102 (2019). [Open Access URL]
Group(s): Goedecker / Project(s): DD1 - A. Grisafi, M. Ceriotti, Incorporating long-range physics in atomic-scale machine learning, The Journal of Chemical Physics 151, 204105 (2019). [Open Access URL]
Dataset on Materials Cloud.
Group(s): Ceriotti / Project(s): DD1 - B. A. Helfrecht, R. Semino, G. Pireddu, S. M. Auerbach, M. Ceriotti, A new kind of atlas of zeolite building blocks, The Journal of Chemical Physics 151, 154112 (2019). [Open Access URL]
Dataset on Materials Cloud.
Group(s): Ceriotti / Project(s): DD1 - T. Karmakar, P. M. Piaggi, M. Parrinello, Molecular Dynamics Simulations of Crystal Nucleation from Solution at Constant Chemical Potential, Journal of Chemical Theory and Computation 15, 6923 (2019). [Open Access URL]
Dataset on Materials Cloud.
Group(s): Parrinello / Project(s): DD1 - J. Zhang, Y. I. Yang, F. Noé, Targeted Adversarial Learning Optimized Sampling, The Journal of Physical Chemistry Letters 10, 5791–5797 (2019). [Open Access URL]
Group(s): Parrinello / Project(s): DD1 - D. Dutta, D. S. De, D. Fan, S. Roy, G. Alfieri, M. Camarda, M. Amsler, J. Lehmann, H. Bartolf, S. Goedecker, T. A. Jung, Evidence for carbon clusters present near thermal gate oxides affecting the electronic band structure in SiC-MOSFET, Applied Physics Letters 115, 101601 (2019). [Open Access URL]
Group(s): Goedecker / Project(s): DD1 - V. Kapil, E. Engel, M. Rossi, M. Ceriotti, Assessment of Approximate Methods for Anharmonic Free Energies, Journal of Chemical Theory and Computation 15, 5845–5857 (2019). [Open Access URL]
Dataset on Materials Cloud.
Group(s): Ceriotti / Project(s): DD1 - L. Bonati, Y. Zhang, M. Parrinello, Neural networks-based variationally enhanced sampling, Proceedings of the National Academy of Science of the USA 116, 17641–17647 (2019). [Open Access URL]
Dataset on Materials Cloud.
Group(s): Parrinello / Project(s): DD1 - D. Han, T. Karmakar, Z. Bjelobrk, J. Gong, M. Parrinello, Solvent-mediated morphology selection of the active pharmaceutical ingredient isoniazid: Experimental and simulation studies, Chemical Engineering Science 204, 320 (2019).
Dataset on Materials Cloud.
Group(s): Parrinello / Project(s): DD1 - Y. Yang, K. U. Lao, D. M. Wilkins, A. Grisafi, M. Ceriotti, R. A. D. Jr., Quantum mechanical static dipole polarizabilities in the QM7b and AlphaML showcase databases, Scientific Data 6, 152 (2019). [Open Access URL]
Dataset on Materials Cloud.
Group(s): Ceriotti / Project(s): DD1 - S. Faraji, S. A. Ghasemi, B. Parsaeifard, S. Goedecker, Surface reconstructions and premelting of the (100) CaF2 surface, Physical Chemistry Chemical Physics 21, 16270 (2019). [Open Access URL]
Dataset on Materials Cloud.
Group(s): Goedecker / Project(s): DD1 - H. Niu, Y. I. Yang, M. Parrinello, Temperature Dependence of Homogeneous Nucleation in Ice, Physical Review Letters 122, 245501 (2019). [Open Access URL]
Dataset on Materials Cloud.
Group(s): Parrinello / Project(s): DD1 - P. M. Piaggi, M. Parrinello, Calculation of phase diagrams in the multithermal-multibaric ensemble, The Journal of Chemical Physics 150, 244119 (2019). [Open Access URL]
Dataset on Materials Cloud.
Group(s): Parrinello / Project(s): DD1 - M. Graužinytė, D. Tomerini, S. Goedecker, J. A. Flores-Livas, A Divalent Path to Enhance p-Type Conductivity in a SnO Transparent Semiconductor, The Journal of Physical Chemistry C 123, 14909–14913 (2019).
Dataset on Materials Cloud.
Group(s): Goedecker / Project(s): DD1 - S. Pérez-Conesa, P. M. Piaggi, M. Parrinello, A local fingerprint for hydrophobicity and hydrophilicity: From methane to peptides, The Journal of Chemical Physics 150, 204103 (2019). [Open Access URL]
Group(s): Parrinello / Project(s): DD1 - Z. Bjelobrk, P. M. Piaggi, T. Weber, T. Karmakar, M. Mazzotti, M. Parrinello, Naphthalene crystal shape prediction from molecular dynamics simulations, CrystEngComm 21, 3280 (2019). [Open Access URL]
Group(s): Parrinello / Project(s): DD1 - B. Meyer, S. Barthel, A. Mace, L. Vannay, B. Guillot, B. Smit, C. Corminboeuf, DORI Reveals the Influence of Noncovalent Interactions on Covalent Bonding Patterns in Molecular Crystals Under Pressure, The Journal of Physical Chemistry Letters 10, 1482–1488 (2019). [Open Access URL]
Dataset on Materials Cloud.
Group(s): Corminboeuf, Smit / Project(s): DD1, DD4 - R. A. Puglisi, S. Caccamo, C. Bongiorno, G. Fisicaro, L. Genovese, S. Goedecker, G. Mannino, A. La Magna, Direct observation of single organic molecules grafted on the surface of a silicon nanowire, Scientific Reports 9, 5647 (2019). [Open Access URL]
Group(s): Goedecker / Project(s): DD1 - M. J. Willatt, F. Musil, M. Ceriotti, Atom-density representations for machine learning, The Journal of Chemical Physics 150, 154110 (2019). [Open Access URL]
Group(s): Ceriotti / Project(s): DD1 - O. Andreussi, N. G. Hörmann, F. Nattino, G. Fisicaro, S. Goedecker, N. Marzari, Solvent-Aware Interfaces in Continuum Solvation, Journal of Chemical Theory and Computation 15, 1996–2009 (2019). [Open Access URL]
Group(s): Goedecker, Marzari / Project(s): DD1, DD3 - J. A. Flores-Livas, R. Sarmiento-Pérez, S. Botti, S. Goedecker, M. A. L. Marques, Rare-earth magnetic nitride perovskites, JPhys Materials 2, 025003 (2019). [Open Access URL]
Dataset on Materials Cloud.
Group(s): Goedecker / Project(s): DD1 - M. Invernizzi, M. Parrinello, Making the best of a bad situation: a multiscale approach to free energy calculation, Journal of Chemical Theory and Computation 15, 2187–2194 (2019). [Open Access URL]
Dataset on Materials Cloud.
Group(s): Parrinello / Project(s): DD1 - V. Kapil, M. Rossi, O. Marsalek, R. Petraglia, Y. Litman, T. Spura, B. Cheng, A. Cuzzocrea, R. H. Meißner, D. M. Wilkins, B. A. Helfrecht, P. Juda, S. P. Bienvenue, W. Fang, J. Kessler, I. Poltavsky, S. Vandenbrande, J. Wieme, C. Corminboeuf, T. D. Kühne, D. E. Manolopoulos, T. E. Markland, J. O. Richardson, A. Tkatchenko, G. A. Tribello, V. V. Speybroeck, M. Ceriotti, i-PI 2.0: A universal force engine for advanced molecular simulations, Computer Physics Communications 236, 214–223 (2019). [Open Access URL]
Dataset on Mendeley.
Group(s): Ceriotti, Corminboeuf / Project(s): DD1 - M. Asgari, R. Semino, P. Schouwink, I. Kochetygov, O. Trukhina, J. D. Tarver, S. Bulut, S. Yang, C. M. Brown, M. Ceriotti, W. L. Queen, An In-Situ Neutron Diffraction and DFT Study of Hydrogen Adsorption in a Sodalite-Type Metal-Organic Framework, Cu-BTTri, European Journal of Inorganic Chemistry 2019, 1147–1154 (2019).
Group(s): Ceriotti, Queen / Project(s): DD1, PP7 - J. Debnath, M. Invernizzi, M. Parrinello, Enhanced sampling of transition states, Journal of Chemical Theory and Computation 15, 2454 (2019). [Open Access URL]
Dataset on Materials Cloud.
Group(s): Parrinello / Project(s): DD1 - P. M. Piaggi, M. Parrinello, Multithermal-multibaric molecular simulations from a variational principle, Physical Review Letters 122, 050601 (2019). [Open Access URL]
Dataset on Materials Cloud.
Group(s): Parrinello / Project(s): DD1 - E. A. Engel, A. Anelli, A. Hofstetter, F. Paruzzo, L. Emsley, M. Ceriotti, A Bayesian approach to NMR crystal structure determination, Physical Chemistry Chemical Physics 21, 23385–23400 (2019). [Open Access URL]
Dataset on Materials Cloud.
Group(s): Ceriotti / Project(s): DD1 - A. Fabrizio, A. Grisafi, B. Meyer, M. Ceriotti, C. Corminboeuf, Electron density learning of non-covalent systems, Chemical Science 10, 9424–9432 (2019). [Open Access URL]
Dataset on Materials Cloud.
Group(s): Ceriotti, Corminboeuf / Project(s): DD1 - M. Graužinytė, S. Botti, M. A. L. Marques, S. Goedecker, J. A. Flores-Livas, Computational acceleration of prospective dopant discovery in cuprous iodide, Physical Chemistry Chemical Physics 21, 18839–18849 (2019). [Open Access URL]
Dataset on Materials Cloud.
Group(s): Goedecker / Project(s): DD1 - A. Grisafi, A. Fabrizio, B. Meyer, D. M. Wilkins, C. Corminboeuf, M. Ceriotti, Transferable Machine-Learning Model of the Electron Density, ACS Central Science 5, 57–64 (2019). [Open Access URL]
Dataset on Materials Cloud.
Group(s): Ceriotti, Corminboeuf / Project(s): DD1 - M. J. Willatt, F. Musil, M. Ceriotti, Feature Optimization for Atomistic Machine Learning Yields a Data-Driven Construction of the Periodic Table of the Elements, Physical Chemistry Chemical Physics 20, 29661–29668 (2018). [Open Access URL]
Group(s): Ceriotti / Project(s): DD1, DD2 - M. Graužinytė, S. Goedecker, J. A. Flores-Livas, Towards bipolar tin monoxide: Revealing unexplored dopants, Physical Review Materials 2, 104604 (2018).
Group(s): Goedecker / Project(s): DD1 - F. M. Paruzzo, A. Hofstetter, F. Musil, S. De, M. Ceriotti, L. Emsley, Chemical shifts in molecular solids by machine learning, Nature Communications 9, 4501 (2018). [Open Access URL]
Dataset on Materials Cloud.
Group(s): Ceriotti / Project(s): DD1 - J. S. C. Kearney, M. Graužinytė, D. Smith, D. Sneed, C. Childs, J. Hinton, C. Park, J. S. Smith, E. Kim, S. D. S. Fitch, A. L. Hector, C. J. Pickard, J. A. Flores-Livas, A. Salamat, Pressure-Tuneable Visible-Range Band Gap in the Ionic Spinel Tin Nitride, Angewandte Chemie International Edition 57, 11623–11628 (2018). [Open Access URL]
Group(s): Goedecker / Project(s): DD1 - J. A. Flores-Livas, M. Graužinytė, L. Boeri, G. Profeta, A. Sanna, Superconductivity in doped polyethylene at high pressure, The European Physical Journal B 91, 176 (2018).
Group(s): Goedecker / Project(s): DD1 - J. A. Flores-Livas, D. Tomerini, M. Amsler, A. Boziki, U. Rothlisberger, S. Goedecker, Emergence of hidden phases of methylammonium lead iodide (CH3NH3PbI3) upon compression, Physical Review Materials 2, 085201 (2018). [Open Access URL]
Dataset on Materials Cloud.
Group(s): Goedecker, Roethlisberger / Project(s): DD1, VP2, HP4 - E. Rucavado, M. Graužinytė, J. A. Flores-Livas, Q. Jeangros, F. Landucci, Y. Lee, T. Koida, S. Goedecker, A. Hessler-Wyser, C. Ballif, M. Morales-Masis, New Route for “Cold-Passivation” of Defects in Tin-Based Oxides, The Journal of Physical Chemistry C 122, 17612–17620 (2018). [Open Access URL]
Group(s): Goedecker / Project(s): DD1 - D. S. De, S. Saha, L. Genovese, S. Goedecker, Influence of an external electric field on the potential-energy surface of alkali-metal-decorated C60, Physical Review A 97, 063401 (2018).
Group(s): Goedecker / Project(s): DD1 - J. Yang, S. De, J. E. Campbell, S. Li, M. Ceriotti, G. M. Day, Large-Scale Computational Screening of Molecular Organic Semiconductors Using Crystal Structure Prediction, Chemistry of Materials 30, 4361–4371 (2018).
Group(s): Ceriotti / Project(s): DD1 - Y. I. Yang, M. Parrinello, Refining Collective Coordinates and Improving Free Energy Representation in Variational Enhanced Sampling, Journal of Chemical Theory and Computation 14, 2889–2894 (2018).
Group(s): Parrinello / Project(s): DD1 - V. Kapil, A. Cuzzocrea, M. Ceriotti, Anisotropy of the Proton Momentum Distribution in Water, The Journal of Physical Chemistry B 122, 6048–6054 (2018). [Open Access URL]
Group(s): Ceriotti / Project(s): DD1 - L. E. Ratcliff, A. Degomme, J. A. Flores-Livas, S. Goedecker, L. Genovese, Affordable and accurate large-scale hybrid-functional calculations on GPU-accelerated supercomputers, Journal of Physics: Condensed Matter 30, 095901 (2018). [Open Access URL]
Group(s): Goedecker / Project(s): DD1 - L. Vannay, B. Meyer, R. Petraglia, G. Sforazzini, M. Ceriotti, C. Corminboeuf, Analyzing Fluxional Molecules Using DORI, Journal of Chemical Theory and Computation 14, 2370–2379 (2018).
Group(s): Ceriotti, Corminboeuf / Project(s): DD1 - L. Bonati, M. Parrinello, Silicon liquid structure and crystal nucleation from ab initio deep metadynamics, Physical Review Letters 121, 265701 (2018). [Open Access URL]
Group(s): Parrinello / Project(s): DD1 - D. Sankar De, J. A. Flores-Livas, S. Saha, L. Genovese, S. Goedecker, Stable structures of exohedrally decorated C60-fullerenes, Carbon 129, 847 (2018). [Open Access URL]
Group(s): Goedecker / Project(s): HP4, DD1 - P. Gasparotto, R. H. Meißner, M. Ceriotti, Recognizing Local and Global Structural Motifs at the Atomic Scale, Journal of Chemical Theory and Computation 14, 486–498 (2018). [Open Access URL]
Group(s): Ceriotti / Project(s): DD1 - G. Gryn'ova, K. Lin, C. Corminboeuf, Read between the Molecules: Computational Insights into Organic Semiconductors, Journal of the American Chemical Society 140, 16370–16386 (2018). [Open Access URL]
Group(s): Corminboeuf / Project(s): DD1 - K. Tarak, P. M. Piaggi, C. Perego, M. Parrinello, A Cannibalistic Approach to Grand Canonical Crystal Growth, Journal of Chemical Theory and Computation 14, 2678–2683 (2018).
Group(s): Parrinello / Project(s): DD1 - D. Mendels, J. McCarty, P. M. Piaggi, M. Parrinello, Searching for Entropically Stabilized Phases: The Case of Silver Iodide, The Journal of Physical Chemistry C 122, 1786–1790 (2018).
Group(s): Parrinello / Project(s): DD1 - B. Meyer, B. Sawatlon, S. Heinen, O. A. von Lilienfeld, C. Corminboeuf, Machine learning meets volcano plots: computational discovery of cross-coupling catalysts, Chemical Science 9, 7069 (2018). [Open Access URL]
Dataset on Materials Cloud.
Group(s): Corminboeuf, von Lilienfeld / Project(s): DD1, INC2 - F. Musil, S. De, J. Yang, J. E. Campbell, G. M. Day, M. Ceriotti, Machine learning for the structure-energy-property landscapes of molecular crystals, Chemical Science 9, 1289–1300 (2018). [Open Access URL]
Group(s): Ceriotti / Project(s): HP5, DD1 - H. Niu, P. M. Piaggi, M. Invernizzi, M. Parrinello, Molecular dynamics simulations of liquid silica crystallisation, Proceedings of the National Academy of Science of the USA 115, 5348–5352 (2018). [Open Access URL]
Group(s): Parrinello / Project(s): DD1 - C. Perego, O. Valsson, M. Parrinello, Chemical potential calculations in non-homogeneous liquids, The Journal of Chemical Physics 149, 072305 (2018). [Open Access URL]
Group(s): Parrinello / Project(s): DD1 - P. M. Piaggi, M. Parrinello, Predicting polymorphism in molecular crystals using orientational entropy, Proceedings of the National Academy of Science of the USA 115, 10251–10256 (2018). [Open Access URL]
Group(s): Parrinello / Project(s): DD1 - O. Valsson and M. Parrinello, Variationally Enhanced Sampling, in Handbook of Materials Modeling : Methods: Theory and Modeling, W. Andreoni and S. Yip, eds. (Springer, Cham, 2018), pp. 1–14.
Group(s): Parrinello / Project(s): DD1 - Y. Wang, O. Valsson, P. Tiwary, M. Parrinello, K. Lindorff-Larsen, Frequency adaptive metadynamics for the calculation of rare-event kinetics, The Journal of Chemical Physics 149, 072309 (2018). [Open Access URL]
Group(s): Parrinello / Project(s): DD1 - Y. I. Yang, H. Niu, M. Parrinello, Combining Metadynamics and Integrated Tempering Sampling, The Journal of Physical Chemistry Letters 9, 6426–6430 (2018). [Open Access URL]
Group(s): Parrinello / Project(s): DD1